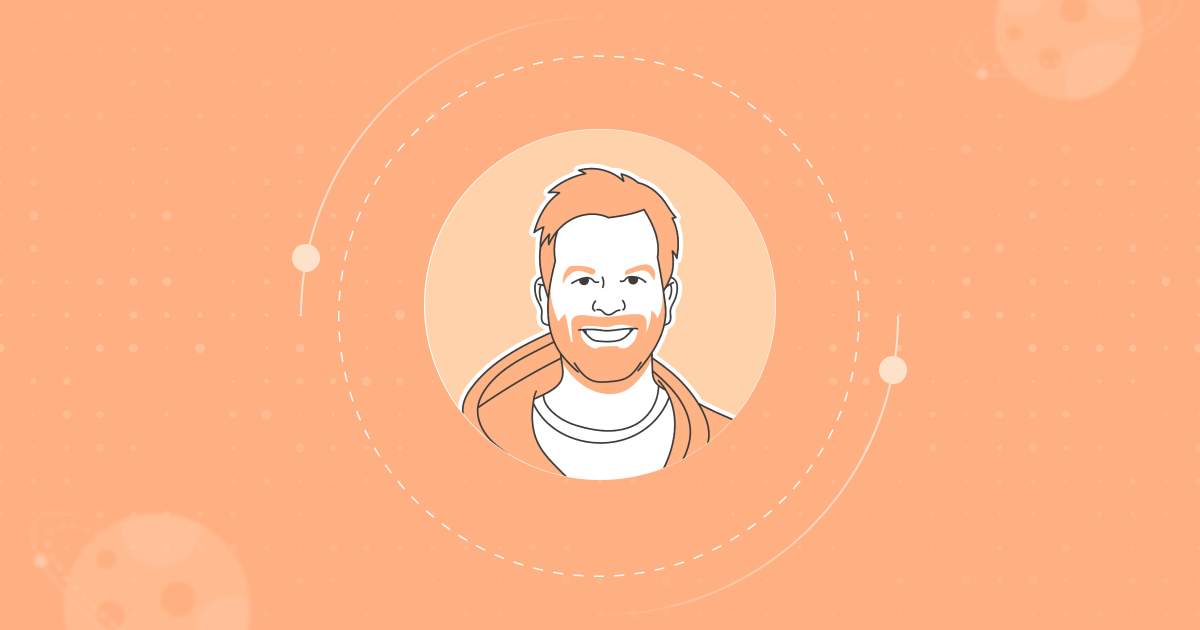
Touch screens have become so ubiquitous, and using them is so intuitive these days that most gadget users probably do not think twice about it. Swipe right if you agree.
But stop for a moment: would you touch another person to communicate if you could speak to them instead? It's faster and easier, plus you hear the emotions and sentiments embedded in the message. We already have Alexa and Siri, voice search on YouTube, and many other ways to use voice for interactions with the technologies. So why is voice not used more widely?
Because making it work smoothly and naturally on par with real-life conversation is not as easy as we would like it to be. That's why we need pioneers like Hannes Heikinheimo, who is working on making voice widespread and similarly accessible to touch.
His first encounter with machine learning was at the department of computer science at Helsinki University of Technology during his Master's studies. By the time Hannes graduated and joined the research group of Professor Heikki Mannila as a Ph.D. student, it became pretty apparent that he was in his element, focusing on pattern mining and clustering for discrete high-dimensional data.
Other works by Hannes include predictive analytics and music recommendation systems at Nokia as a senior data scientist, followed by research on human-in-the-loop machine learning algorithms at Aalto University such as "The Crowd-Median Algorithm" and "Crowdsourced Nonparametric Density Estimation Using Relative Distances" (check out his Scholar page). At Rovio he led the development work of Rovio's multi-terabyte-a-day in-game analytics pipeline.
Next came Reaktor, and after consulting large gaming and media companies for a while Hannes shifted his focus back to machine learning and became one of the four NLP engineers working on the first version of Apple's Siri for the Finnish language. At the same time, there were very interesting things happening in voice: in academia, the first human parity results in automatic speech recognition, while Amazon Alexa ushered in a new wave of voice applications.
There could not have been a more perfect time to start a company. The stars aligned, and Speechly was born.
Voice chat moderation in action.
Voice is the new touch
The company's biggest aspiration is to "do for voice what iPhone did for touch".
While voice user interfaces have gained popularity, they are still, in many ways, very clumsy and slow to use. They don't support hesitation, self-correction, or barge-in. Speechly sees the situation as a bit similar to the touch modality before the iPhone when the touch screen experience was still rather clumsy. Some might remember the pre-iPhone touchscreen being very slow.
Since then, the team has noticed that voice technology isn't just about providing another user interface. During their time at Y Combinator, Speechly got pulled into the voice moderation space, and now it is set to solve the voice moderation problem. Their team's capability to deploy their speech recognition on-device is critical, in addition to their speed and adaptation capabilities.
"Major gaming studios like Riot Games, Roblox and Sony are recording voice chats for moderation, but the tools for content moderation today typically suffer from low accuracy, high cost, and high latency. A new technical approach is needed to fill the voice chat moderation gap." - Otto Söderlund, Speechly
On a smaller scale, Speechly is focused on combining extreme cost efficiency with speed and extreme accuracy in speech recognition. In such a way, the team hopes to improve the quality of interaction between humans as well as humans and computers.
"For me, it's about being able to help people to solve relevant and painful problems to the extent that they are willing to pay for the solution." - Hannes Heikinheimo, founder of Speechly.
Transcription in action.
What's next?
Hannes believes that the next major advances in speech recognition will involve synthetic data and unsupervised models around audio.
If you feel like this is a challenge you would like to pioneer as a machine learning engineer, Hannes believes you should follow Andrew Ng's advice: read a lot of papers, replicate other people's results, and don't be afraid to do dirty work. For instance, collecting data through crowdsourcing, scraping or doing error analysis by looking at examples one at a time. All of the glamorous results in machine learning result from doing a lot of dirty work.
If you want to know more about Speechly, be sure to check out their website and follow them on Twitter or LinkedIn. To learn more about Hannes, follow his LinkedIn profile as well.