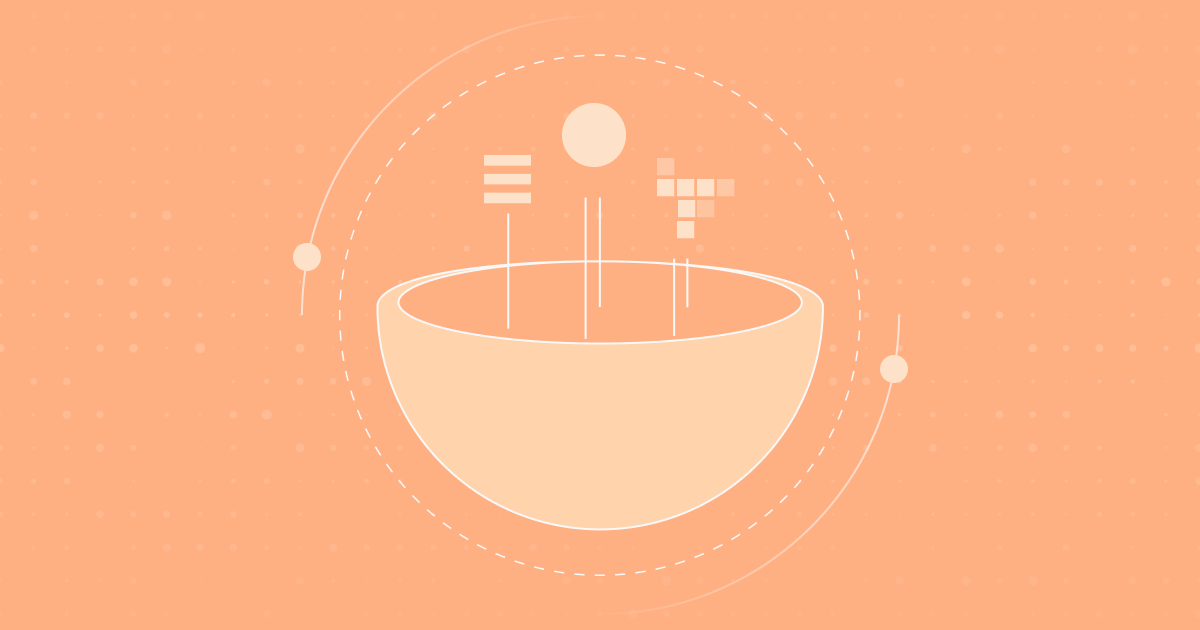
By 2030, artificial intelligence will contribute up to $15.7 trillion to the global economy (PwC). Today, machine learning already enables companies to build products that disrupt their existing market or create entirely new ones. The power of machine learning is the ability to solve problems that are too complex for us to solve with logic defined by hand. Today, these tasks are handled mainly by experts – such as radiologists interpreting x-ray images – or ignored because of the impracticality.
With great power comes great responsibility. No, I'm not talking about making sure AI doesn't take over the world. Instead, I'm talking about the responsibility of handling the uncertainty that ML introduces. ML algorithms cannot be defined and designed the same way as traditional software. As a result, there is uncertainty about whether a working solution can be developed, how well it'll perform in the real world, and whether it's all ethical and desirable.
Still, there is hardly any sector or industry that will not be affected by machine learning and AI. For some companies, the stakes are even higher as ML will become the core of their product. We've named that category "ML Peioneers" as they are early movers and have to invent the playbook as they go.
We are lucky to work with several of these pioneers at Valohai. Below I've outlined three building blocks that we see in each of them. Consider these the foundation for an ML Pioneer.
Building a solid foundation with the 3Ps
1. People
No organization should undermine the power of people. Whatever you do, talent is essential. This is even more critical when it comes to machine learning. The importance of cross-functionality cannot be overstated. In addition to data scientists, successful teams include engineers who can help deploy and automate, product managers who help align goals and expectations, and subject matter experts who understand the real-world context. A shared understanding of what machine learning is capable of (ML is not magic) and shared goals are also core to success.
Firms with the right talent will rule the AI world. The race for dominance in AI has also led to a shortage of data science and engineering talent as companies are ready to hire the best. People must be the center of everything you do!
2. Processes
Another bedrock of an ML Pioneer is your standard practices. Your talent must be mission-oriented, do things in the right way, and maintain a consensus towards building for the long term. Indeed, AI innovations that will shape the world will be sustainable, resilient, and stand the test of time.
Like processes in software engineering, there is no one-size-fits-all approach. How often you release, how you define done, or how you document solutions are all things that'll have to be built into how your team works together. You must also ensure that your team does things responsibly and comply with privacy, governance, and ethics requirements. Ideally, you'll get started with small, well-defined ML projects that have a clear pathway to production, and along the way, you build yourself a process to get on to bigger and better things.
3. Platforms
Technologies and infrastructures must be in place to help you achieve your goal. These platforms constitute the bridge that supports people and processes. They breed consistency and help you avoid reinventing the wheel. Platforms are essential for sharing and reusing Heureka-moments in your AI journey. For instance, replicating and repurposing existing pipelines to serve multiple problems is much more efficient than starting from scratch every time.
Unlike people and processes, you do not have to build all the platforms and technologies you need to become a pioneer. Instead, you can partner with reliable solution providers to streamline your processes and promote your mission.
Case Studies: Tying the 3Ps together
Next we will explore two case studies of disruptive firms that have taken advantage of the 3Ps to become ML Pioneers in their domains.
Preligens
Preligens understands the power of people in its mission to build cutting-edge software for intelligence analysts to monitor strategic sites and track objects. The AI-technology pioneer assembled one of the largest deep learning-focused teams in Europe to execute its plan. It currently has over 150 team members, including the best-in-class R&D team and more than 50 data scientists. Preligens is also leveraging Valohai's expertise to minimize the time its talent spends on managing model training infrastructure. The firm's operations follow an established series of actions and processes in its quest to build AI solutions to international security challenges. Preligens combines computer vision and machine learning to build a seamless AI security solution. The software draws from a multitude of data sources, including satellite imagery (IMINT), signal intelligence (ELINT), and open-source intelligence (OSINT), to provide the best picture of the situation on the ground. Preligens has also developed a proprietary framework on top of TensorFlow to industrialize R&D and model creation. The framework streamlines all the steps between experimentation and production. However, Preligens needed additional capabilities to industrialize MLOps, machine deployment, and job orchestration. For this, it chose the Valohai MLOps platform. Valohai provides Preligens with a shared platform, managed infrastructure, and integratable architecture. Valohai automatically tracks every experiment run on the platform, storing all the metadata and artifacts. Within the Valohai platform, previous works are shared between the data scientists such that new team members can access the experiment history and understand how models have evolved.
KONUX
KONUX is another great case study. The firm has a strong team of experts and highly motivated people focused on transforming railway operations for a sustainable future. The company's core values include grit, ingenuity, and credibility. KONUX also has well-defined working processes and strategies to implement its goal of combining IIoT devices and machine learning algorithms to deliver software-as-a-service solutions for operation, monitoring, and maintenance process automation. The IIoT devices are placed along the track to measure vibration from passing trains. At the same time, intelligent analytics are used to detect and identify trains and railcars on the rail from the sensor data and alert to any abnormalities. All KONUX processes and actions are focused on helping railway operators dispatch maintenance crews to ensure safety, improve availability and prolong the lifespan of the existing infrastructure. Like Preligens and other disruptive AI solution providers, KONUX also chose Valohai at its MLOps platform. The data science team uses Valohai to continuously train production models that provide insights within their SaaS product. In addition to that, they also run exploratory research (called moonshots) on Valohai. Each team member can spend 15% of their time on moonshots to find breakthroughs.
Wrap up
The 3Ps presented here are large and fuzzy concepts, and there isn't a single best way to develop each – although we have a clear favorite for the platform part. People, Processes and Platforms are three areas that leaders should focus on developing in tandem. Unsupported team members will be ineffective and platforms alone can't provide value, but with the right balance of the 3Ps, your team can build something the world hasn't yet seen.
You can read more about Preligens and KONUX in our AI trailblazers booklet.